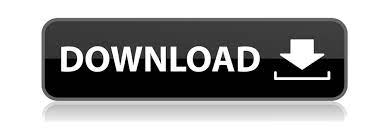

SparkR automatically infers the schema from the CSV file.

SparkR supports reading CSV, JSON, text, and Parquet filesĭiamondsDF <- read.df("/databricks-datasets/Rdatasets/data-001/csv/ggplot2/diamonds.csv", source = "csv", header="true", inferSchema = "true") This method takes the path for the file to load and the type of data source.
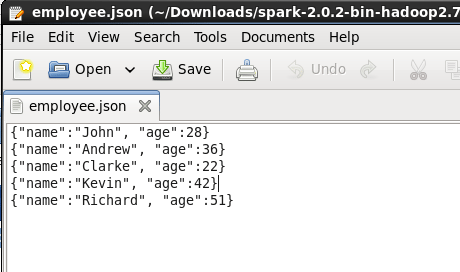
The general method for creating a DataFrame from a data source is read.df. # Displays the content of the DataFrame to stdout
Could not find function sparkr.session in sparkr code#
You can see examples of this in the code snippet bellow.įor more examples, see createDataFrame. Like most other SparkR functions, createDataFrame Specifically we can use createDataFrame and pass in the local Rĭata.frame to create a SparkDataFrame. The simplest way to create a DataFrame is to convert a local R ame into a You can create a DataFrame from a local R ame, from a data source, or using a Spark SQL query. For an example, see Create and run a spark-submit job for R scripts. You can run scripts that use SparkR on Azure Databricks as spark-submit jobs, with minor code modifications. The SparkR session is already configured, and all SparkR functions will talk to your attached cluster using the existing session. To use SparkR you can call library(SparkR) in your notebooks. For Spark 2.2 and above, notebooks no longer import SparkR by default because SparkR functions were conflicting with similarly named functions from other popular packages.For Spark 2.0 and above, you do not need to explicitly pass a sqlContext object to every function call.SparkR also supports distributed machine learning using MLlib. SparkR is an R package that provides a light-weight frontend to use Apache Spark from R.
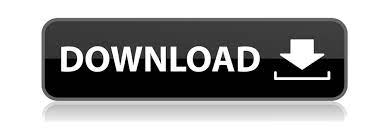